Big Data & Machine Learning
- Primavera Fisogni
- Jan 22, 2023
- 8 min read
Updated: Jan 27, 2023
The Onlife age, a word coined in 2015 by Oxford scholar and Italian philosopher Luciano Floridi has changed our lives: the Covid-19 pandemic has speeded up several changes, in a flood of digital data. It's time to have a better understanding of the two main engines of such a transformation.
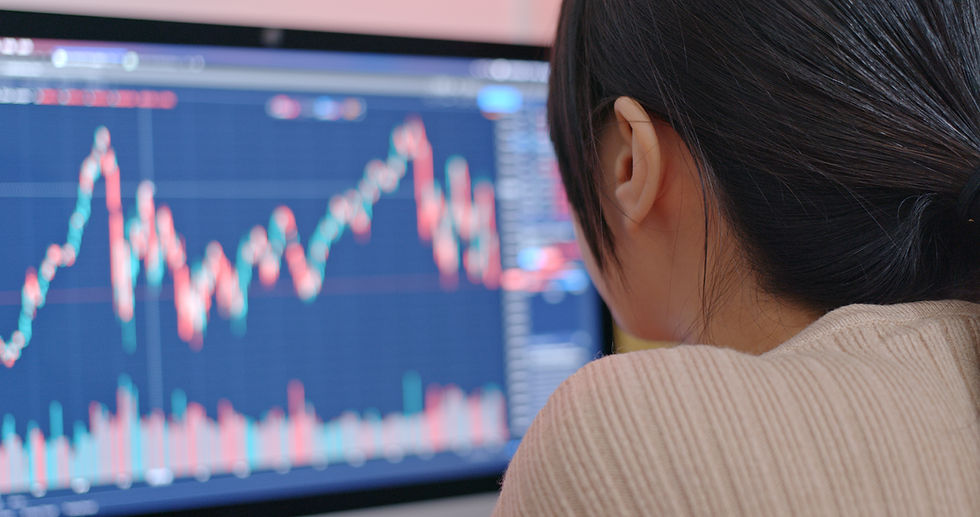
By Primavera Fisogni
Big Data and Machine Learning are terms that are more and more part of our daily lives. No surprise that both these digital topics are driving the Forth Revolution. It's a honour and a pleasure, as the editor of Rekh Magazine, to have an interview with professor John Wang, the curator of the Encyclopedia of Data Science and Machine Learning, published by IGI Global, in the US, to which I also joined as a contributor.
Big Data is a dynamic reality that continues to evolve. Why is the demand for knowledge in the field increasing?
Big data refers to the large and complex datasets that organizations generate and collect, often from a variety of sources. These datasets can be difficult to process and analyze using traditional data processing techniques, and as a result, there is a growing demand for tools and technologies that can help organizations make sense of this data. The demand for knowledge in the field of big data is increasing because organizations are recognizing the value of being able to effectively collect, store, process, and analyze this data. By doing so, they can gain insights that can help them make better decisions, improve their operations, and gain a competitive advantage. Additionally, as more and more organizations generate and collect large amounts of data, there is a need for skilled professionals who can help them manage and make sense of this data. This is driving demand for people with knowledge and expertise in the field of big data. Big Data vs. Machine Learning: How do they differ and relate?
Big data and machine learning are related, but they are not the same thing. Big data refers to large and complex datasets that organizations generate and collect, often from a variety of sources. These datasets can be difficult to process and analyze using traditional data processing techniques. Machine learning, on the other hand, is a type of artificial intelligence that allows systems to learn and improve their performance over time without being explicitly programmed. One way that big data and machine learning are related is that machine learning algorithms can be used to analyze and make sense of big data. By training machine learning models on large datasets, organizations can gain insights and make predictions that would be difficult or impossible to obtain using traditional data processing techniques. However, it is important to note that machine learning is just one way to analyze big data, and it is not always the most appropriate solution. There are other techniques and tools that can be used to process and analyze big data, depending on the specific needs and goals of an organization.
Theword Big data refers to large and complex datasets that organizations generate and collect, often from a variety of sources. These datasets can be difficult to process and analyze using traditional data processing techniques. Machine learning, on the other hand, is a type of artificial intelligence that allows systems to learn and improve their performance over time without being explicitly programmed.

(Wix pic)
How can they (Big Data and Machine Learning) be used together? Big data and machine learning can be used together in a number of ways. Here are a few examples: Training machine learning models on big data: Machine learning algorithms can be trained on large datasets in order to make predictions or identify patterns that would be difficult or impossible to detect using traditional data processing techniques. Analyzing big data to improve machine learning models: Organizations can use big data to improve the performance of their machine learning models by using it to fine-tune model hyperparameters, detect bias in the training data, and more. Using machine learning to process and analyze big data: Machine learning can be used to process and analyze big data in real-time, allowing organizations to make quick decisions based on the insights they gain from the data.
Building machine learning models to generate insights from big data: Organizations can use machine learning to build models that automatically generate insights from big data, such as identifying trends or predicting future outcomes. Overall, the combination of big data and machine learning can help organizations gain a deeper understanding of their data and make better informed decisions.
Would you please sketch the state-of-the-art research in the areas of data science, machine learning, and data mining?
Certainly! Here is a high-level overview of the state-of-the-art in the fields of data science, machine learning, and data mining:
Data Science: Data science is a multidisciplinary field that uses scientific methods, processes, algorithms, and systems to extract knowledge and insights from structured and unstructured data. The goal of data science is to uncover hidden patterns, trends, and relationships in data, and to use these insights to make informed decisions and predictions.
Machine Learning: Machine learning is a subset of artificial intelligence that involves the use of algorithms and statistical models to enable computers to learn from data, without being explicitly programmed. Machine learning techniques are used to build predictive models and make data-driven decisions.
Data Mining: Data mining is the process of discovering patterns and relationships in large datasets. It involves the use of machine learning algorithms and statistical analysis to identify trends and make predictions. Data mining can be used to discover patterns in data that can be used to make decisions and predictions.
Some of the current research trends in these fields include:
Deep Learning: Deep learning is a type of machine learning that involves the use of neural networks to learn from data. Deep learning algorithms are particularly well-suited for analyzing large, complex datasets, and have been applied to a variety of tasks including image and speech recognition, natural language processing, and machine translation.
Big Data: The amount of data being generated and collected has grown exponentially in recent years, leading to the development of new technologies and techniques for storing, processing, and analyzing large datasets. These technologies, collectively known as big data, are being used to extract insights from data at scale.
Explainable AI: There is growing interest in developing artificial intelligence systems that are transparent and interpretable, so that their decisions and predictions can be understood by humans. This is particularly important for applications in which the stakes are high, such as healthcare and finance.
Reinforcement Learning: Reinforcement learning is a type of machine learning in which an agent learns to interact with its environment in order to maximize a reward. It has been used to train computer programs to play games and control robots, and has the potential to be applied to a wide range of real-world problems.

Big data and machine learning are driving the Fourth Industrial Revolution (Wix pic).
Machine learning can be used to process and analyze big data in real-time, allowing organizations to make quick decisions based on the insights they gain from the data.
Big data technologies have been adopted by various entities to expedite the decision-making process and make it more effective and efficient. On the other hand, what are the major limits and critical issues that emerge? There are several limitations and critical issues that can emerge when using big data technologies to make decisions:
Quality of data: One major issue is the quality of the data being used. If the data is incomplete, inaccurate, or biased, it can lead to flawed or incorrect decisions. Privacy concerns: The use of big data can raise privacy concerns, as large amounts of personal data are often collected and analyzed. This can be a particular concern in cases where sensitive information is involved, such as medical records or financial data. Ethical considerations: The use of big data can also raise ethical concerns, such as whether it is fair to use data in certain ways or to make decisions that could have a negative impact on certain groups of people. Technical challenges: Working with big data can also present technical challenges, such as the need for specialized infrastructure and software, and the need for skilled personnel to manage and analyze the data. Costs: The costs associated with implementing and maintaining a big data system can also be a limitation, as it can be expensive to set up and run such a system. Has the pandemic accelerated the need for the understanding / the use of Big Data?
The COVID-19 pandemic has certainly highlighted the importance of data and data analytics in decision-making. Governments, health organizations, and businesses have all turned to data to inform their responses to the pandemic and to make decisions about how to mitigate its impact. For example, data has been used to track the spread of the virus, to understand the effectiveness of different interventions, and to inform the allocation of resources. In this sense, the pandemic has likely accelerated the need for understanding and using big data in order to make informed, data-driven decisions.

You are the editor of the Encyclopedia of Big Data by IGI GLOBAL. How were to link together so many different contributors? And, finally, what valuable paths – also unexpected – have come out from this deep, multifaceted domain?
I can provide some general information about how encyclopedias are typically edited and organized. Typically, an editor of an encyclopedia will work with a team of contributors, who are experts in their respective fields. The editor will coordinate with these contributors to ensure that the content of the encyclopedia is accurate, up-to-date, and covers a wide range of topics. The editor may also work with a publisher to ensure that the final product meets the necessary standards and is ready for distribution. One of the key challenges in editing an encyclopedia is organizing and linking together the various contributions in a logical and coherent manner. This can involve creating a clear and consistent structure for the content, as well as ensuring that there are appropriate cross-references and links between related topics. In terms of valuable paths that may emerge from the editing process, it is not uncommon for unexpected connections and insights to emerge as the editor works with the contributors and reviews the content. The process of organizing and synthesizing information from multiple sources can lead to new understanding and perspectives on the subject matter.
© Rekh Magazine

John Wang
John Wang is a professor in the Department of Information Management and Business Analytics at Montclair State University, USA. Having received a scholarship award, he came to the USA and completed his PhD in operations research from Temple University. Due to his extraordinary contributions beyond a tenured full professor, Dr. Wang has been honored with two special range adjustments in 2006 and 2009, respectively. He has published over 100 refereed papers and seventeen books. He has also developed several computer software programs based on his research findings. He serves as Editor-in-Chief for ten Scopus-indexed journals, such as Int. J. of Business Analytics, Int. J. of Information Systems and Supply Chain Management, Int. J. of Information Systems in the Service Sector, Int. J. of Applied Management, Int. J. of Information and Decision Sciences, Int. J. of Data Mining, Modelling and Management, etc. He is the Editor of Encyclopedia of Business Analytics and Optimization (five volumes), Data Warehousing and Mining: Concepts, Methodologies, Tools, and Applications (six-volume) and the Editor of the Encyclopedia of Data Warehousing and Mining, 1st (two-volume) and 2nd (four-volume). His long-term research goal is on the cooperation of operations research, data mining and cybernetics.
Comentarios